One of our customers, a leading supplier of critical parts using additive manufacturing (AM), faced a critical challenge in their quality assurance workflow. Previously, one of their quality assurance requirements involved an engineer to manually review every image of each build. To accomplish this, the team would consolidate thousands of layer images into a video and watch it closely, manually searching for any signs of failures or defects part of the print quality check.
Figure1: A 30-minute build review video, shown here in a time-lapse format,illustrates how thousands of layer images are consolidated for manual engineer review.
This method posed several major problems:
- Human Limitations: Engineers were expected to catch subtle anomalies in videos that could be as long as an hour. This approach was both mentally exhausting and unreliable. While larger issues could be identified easily, if the engineer remained fully focused, confidence in consistently catching every anomaly was low, and this limitation was well understood.
- Change Blindness: It is known that when reviewing thousands of layer images in rapid succession, engineers experience change blindness, a psychological phenomenon where significant visual changes go unnoticed (source: Wikipedia). This effect makes it easy to miss subtle but critical anomalies during manual review. The repetitive nature of scanning these images, combined with the fast pace and potential distractions, further lowers the likelihood of catching every issue, even for highly experienced engineers.
- Time-Intensive: Reviewing a 2,000-layer build would take up to an hour of an engineer’s time. With 20 systems running one and a half builds per week, the annual review time was costing the company around $75,000 in labor alone.
- Artificial Intelligence (AI) Restrictions: In AM, many are beginning to rely on AI models and machine learning to identify anomalies. These models come with their own issues:
- Visibility Constraints: AI models can't detect defects that aren't visible in the image. For example, Phase3D’s work with the U.S Air Force identified that anomalies under 50 microns, known to cause porosity, are often not visible to a standard camera due to challenging lighting conditions in the machine. Despite this, engineers continue to rely on low-resolution cameras to spot shadows that are barely visible to the human eye.
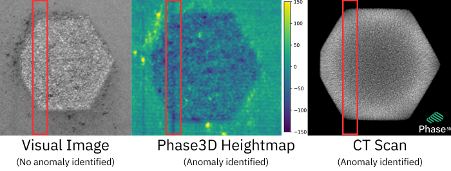
- Dependence on Training Data: AI models are only as good as the data they're trained on, which can vary significantly based on the machine, material, and, in the case of melted anomalies, specific printing parameters. This makes consistency and reliability a major concern for anyone using AI to review visual images. Even the best trained commercial models for image detection make mistakes and miss items.
At Phase3D, we recognize that AI is a powerful tool, but we believe it is not sufficient on its own for quality assurance. To our knowledge, no manufacturing facility relies exclusively on visual cameras to assess a part’s performance. Accurate, unit-level data is essential for conducting reliable inspections.
The Fringe Inspection™ Solution:
Phase3D provides a fundamentally different approach with Fringe Inspection™, replacing subjective visual reviews and unreliable AI detection with objective, layer-by-layer height map measurements. This paradigm shift in in-situ technologies enables:
- Quantified Anomaly Detection: Each layer of the build is measured using Fringe projection, detecting anomalies through objective, unit-based measurements rather than relying on AI models or a human to identify minor variations in the build process.
- Instant Feedback via Quality Charts: Fringe Inspection™ automatically generates a Quality Chart showing problem regions by layer, giving engineers immediate notifications into issues, based not only detection but also severity.
- 3D Visualization: Instead of watching videos, engineers can now explore a real-time 3D visualization of the build to instantly understand the size, depth, and location of defects.
This data-driven method shifts the customer's process from guesswork and intuition to measurable and actionable insights.

The Results:
- Lower Review Time: What once took engineers 60 minutes per build now takes just 5 minutes with Fringe Inspection™.
- Cost Reduction: The facility reduced its annual build review costs from $75,000 to under $7,500, saving over 90%.
- Improved Quality Assurance: With Fringe Inspection™, the customer no longer relies on either human vision or blackbox AI. They now identify build issues objectively and in real-time, empowering engineers to make confident and informed decisions.
- Trusted for Critical Parts: Perhaps most importantly, this enhanced level of quality assurance is crucial for a supplier of critical parts in AM. Fringe Inspection™ has given the customer a reliable method of ensuring their continued success.
Fringe Inspection™ transformed this customer’s quality assurance process, reducing costs, increasing efficiency,and most importantly, giving them confidence in the quality of every build. In an industry where failure is not an option, they now have a tool they can trust.
Due to confidentiality, this case study is a representation of real events. No confidential figures were used, and all data is recreated based on reported findings.